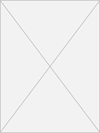
SharkTank Deal Prediction: Dataset and Computational Model
Presenter(s)
Thomas Sherk
Files
Description
SharkTank is a television show where start-ups pitch their idea to a panel of five investors (sharks) in hopes of striking a deal in the form of equity or royalties for money and other business perks. Since its inception, SharkTank has been a center of discussion and analysis for fans, statisticians, and business people alike in hopes of cracking the code to the start-up world and figuring out the next big ‘thing’. Most of these discussions and analyses have come in the form of blogs, articles, and academic research. However, there has been a lack of complete datasets and application of computational models for further analysis. In this project, we investigate factors that play into the SharkTank deal. To this end, we first collect a new dataset, SharkTank Deal Dataset (STDD), by combining data from multiple public sources. The dataset includes descriptive features of each start-up and episode such as product category, team composition, valuation, equity offering, specific sharks that appear on that episode, and state origin. For the computational model, we propose the non-negative least square regression with regularization term to predict whether a start-up strikes a deal with a shark. We conduct experiments to demonstrate the superiority of our model over the baselines, namely, neural network, non-negative least square, ridge regression.
Publication Date
4-24-2019
Project Designation
Course Project
Primary Advisor
Van Tam Nguyen
Primary Advisor's Department
Computer Science
Keywords
Stander Symposium project
Recommended Citation
"SharkTank Deal Prediction: Dataset and Computational Model" (2019). Stander Symposium Projects. 1524.
https://ecommons.udayton.edu/stander_posters/1524
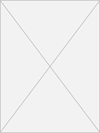
Comments
This poster reflects research conducted as part of a course project designed to give students experience in the research process.