Presenter(s)
Redha Ali
Files
Download Project (578 KB)
Description
Skin cancer is one of cancer type that has a significant impact on society in the United States and across the world. Recently, several Computer-Aided Diagnosis (CAD) system papers have been presented. However, there is still an opportunity for further development in the accuracy of its diagnosis. In this research, we propose an algorithm for skin cancer segmentation and classification at a more treatable stage. Our current approach is computationally efficient and combines information from both deep learning and handcrafted features. Our system creates robust hybrid features that have a stronger discrimination ability than single method features. These features are used as inputs to a decision-making model that is based on a Support Vector Machine (SVM) classifier. Our results evaluated online validation and test databases. Our score was 0.841 on the validation dataset and 0.701 on the test dataset for the classification task. We participated in the ISIC Challenge 2018, being ranked 59th for disease classification and 85th for skin lesion segmentation out of 141 methods listed on the competition leaderboard. These statistics do not include the rankings of the groups who did not qualify for the leaderboard. Also, it is important to note that many of the successful methods that were ranked highly used additional external data for training. The ISIC 2018 competition does not provide the external data that they used. We only utilized the competition which provided data for training.
Publication Date
4-24-2019
Project Designation
Graduate Research
Primary Advisor
Russell C. Hardie
Primary Advisor's Department
Electrical and Computer Engineering
Keywords
Stander Symposium project
Recommended Citation
"Skin Lesion Segmentation and Classification using Deep Learning and Handcrafted Features" (2019). Stander Symposium Projects. 1616.
https://ecommons.udayton.edu/stander_posters/1616
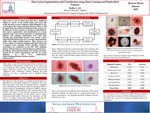