Presenter(s)
Zhiyuan Xie
Files
Download Project (2.4 MB)
Description
The increased temperature over the past decades has affected the health, dynamics, and processes of the alpine-type glaciers. To understand these glacier changes, we used Landsat satellite images, digital elevation model (DEM), DEM-derived land-surface and drainage basin parameters to develop a deep-learning algorithm to map glaciers. The alpine glacier includes the debris-covered glacier (DCG) in the accumulation zone and snow-covered glacier (SCG) in the ablation zone. For this work, we take advantage of the deep-learning algorithm, GlacierNet, which we have already developed to map debris cover ablation zone. The architecture of GlacierNet is based on the commonly used feed-forward neural network – convolutional neural network (CNN). The GlacierNet exploits the spatial pattern present in the debris cover ablation zone; however, the SCG has less distinguishable features when compared with the snow present inside and outside the glacier accumulation zone. Therefore, we consider each glacier as a drainage basin and utilize the drainage basin algorithm to accurately classify the snow and SCG by using the GlacierNet generated DCG as the reference region. Our innovative approach has been successfully applied to selected glaciers in Karakoram and Nepal Himalaya, and the preliminary results indicate high accuracy. This is a major step in developing a fully automated and worldwide applicable methodology for glacier mapping.
Publication Date
4-22-2020
Project Designation
Graduate Research
Primary Advisor
Umesh K. Haritashya, Vijayan K. Asari
Primary Advisor's Department
Geology
Keywords
Stander Symposium project, College of Arts and Sciences
United Nations Sustainable Development Goals
Climate Action
Recommended Citation
"Mapping both accumulation and ablation zone of the glacier using deep-learning" (2020). Stander Symposium Projects. 1804.
https://ecommons.udayton.edu/stander_posters/1804
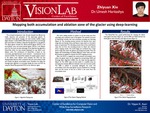
Comments
This presentation was given live via Zoom at 4:00 p.m. (Eastern Time) on Wednesday, April 22.