Presenter(s)
Sai Babu Arigela
Files
Download Project (1.4 MB)
Description
Most of the image/video processing and computer vision applications depend on the high quality image frames. Given a low resolution input, the proposed method uses multi-scale local directional Fourier phase features to adaptively learn a regression kernel based on local covariance to estimate the high resolution image. This method uses image features to learn the local covariance from geometric similarity between low resolution image and its high resolution counterpart. For each patch in the neighbourhood, we estimate four directional variances in three different scales to adapt the interpolated pixels. We use a non parametric kernel regression to learn the characteristics of local directional edge features. The Gaussian steering kernel which has the capability to elongate, rotate and scale along the edge regions is used. The parameters of elongation, rotation and scale are estimated automatically from the image local region. We apply these weights to estimate the interpolated pixels to get the high resolution image. The experimental results show that the proposed algorithm performs better than other state of the art techniques especially at higher resolution scales. This can be applied to improve the performance of object classification system on wide area motion imagery.
Publication Date
4-17-2013
Project Designation
Graduate Research
Primary Advisor
Vijayan K. Asari
Primary Advisor's Department
Electrical and Computer Engineering
Keywords
Stander Symposium project
Recommended Citation
"Multi-Scale Local Fourier Phase Based Feature Learning for Single Image Super Resolution" (2013). Stander Symposium Projects. 201.
https://ecommons.udayton.edu/stander_posters/201
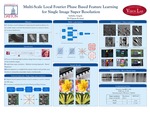