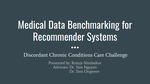
Medical Data Benchmarking for Recommender Systems
Presenter(s)
Rutuja Rajendra Nimbalkar
Files
Download Project
Description
Patients with DCCs often experience multiple obstacles when prioritizing treatment plans and prescriptions. Discordant Chronic Comorbidities (DCCs) are health conditions in which patients have multiple, often unrelated, chronic illnesses that may need to be addressed concurrently but may also be associated with conflicting treatment instructions. Various machine learning (ML) or deep learning (DL) algorithms can provide treatment recommendations and identify drug interactions for patients with DCCs. However, to the best of our knowledge, we have yet to see a good documentation of how conflicting recommendations and evolving patient’s needs could be addressed using machine learning techniques. Further, there are no recommendations for algorithms situations for addressing these complex needs that patients with DCCs experience. And yet, the effective support of DCCs requires decisions aids that capture patients' concerns and preferences upfront, before suggesting a prescription recommendation. In this research, we first collected a data set consisting of the patient with DCCs concerns and prescription preference for each of their medical conditions (e.g., type 2 diabetes, arthritis, and/or depression). We then reviewed studies, models, and algorithms and tested some of these algorithms using a set of criteria with our dataset. And to set benchmark outcomes that can reveal the suitable algorithms, parameters, and testing criteria. Specifically, our research investigates four algorithms for identifying effective and efficient predictions of DCCs prescriptions, while taking into account patients' concerns and drug interactions. Among state-of-the-art supervised ML algorithms, Support Vector Machine (SVM) achieves the best performance. The best algorithms then integrated and deployed in the mobile application interface for user engagement and further evaluations.
Publication Date
4-20-2022
Project Designation
Course Project
Primary Advisor
Van Tam Nguyen, Tom Ongwere
Primary Advisor's Department
Computer Science
Keywords
Stander Symposium project, College of Arts and Sciences
United Nations Sustainable Development Goals
Good Health and Well-Being; Industry, Innovation, and Infrastructure
Recommended Citation
"Medical Data Benchmarking for Recommender Systems" (2022). Stander Symposium Projects. 2469.
https://ecommons.udayton.edu/stander_posters/2469
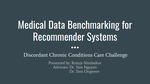
Comments
Presentation: 9:40 a.m.-10:00 a.m., LTC Forum
This project reflects research conducted as part of a course project designed to give students experience in the research process.
Course: CPS 595