Presenter(s)
Alison Hardie
Files
Download Project (3.5 MB)
Description
Glacial lakes provide insight into the melting rates of glaciers; thus, the ability to detect and map them opens possibilities for improved monitoring of the changing size of glacial lakes. An accurate automated method for glacial lake segmentation would provide the means to perform constant monitoring without the need for tedious manual labeling. This work utilizes a deep learning approach using semantic segmentation in MATLAB with convolutional neural networks (CNNs) to automatically detect and map glacial lakes. This work can be used to produce quick estimates of lake areas in order to monitor changes in their size. The network inputs include Landsat 8 spectral bands, ASTER Global Digital Elevation Model V003, which is processed into geomorphic features as an additional input into the network. Glacier outline data is sourced from the Global Land Ice Measurements from Space (GLIMS) database; this data is processed into a glacier distance band and fed into the network. Lake polygon data is sourced from the Annual 30m Dataset for glacial lakes in High Mountain Asia from 2008 to 2017. These labels were loaded and the boundaries were reduced to remove edge and mixed pixels from the training data. The CNN used is DeepLab v3+ with the Resnet 18 backbone. This presentation will include a detailed concept of deep-learning based automated lake mapping and performance analysis, including quantitative result metrics and network output lake boundaries.
Publication Date
4-19-2023
Project Designation
Independent Research
Primary Advisor
Umesh Haritashya
Primary Advisor's Department
Geology
Keywords
Stander Symposium, College of Arts and Sciences
Institutional Learning Goals
Scholarship; Critical Evaluation of Our Times
Recommended Citation
"Utilizing Deep Learning Methods and Semantic Segmentation to Detect and Map Glacial Lakes in the Central Himalayas" (2023). Stander Symposium Projects. 3026.
https://ecommons.udayton.edu/stander_posters/3026
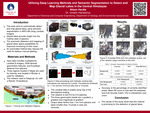
Comments
Presentation: 1:15-2:30 p.m., Kennedy Union Ballroom