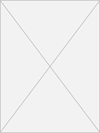
An Efficient Leaf Recognition Based Approach for Plant Classification Using Machine Vision Strategy
Presenter(s)
Redha Ali, Russell C Hardie
Files
Description
The identification of plants is very important component of workflows in plant ecological research. Therefore, in this work, we are developing a novel automatic leaf recognition for plant classification. Unlike the existing techniques, our approach is efficient, fast, and simple. The proposed method is based on machine vision strategy that employs two main processing stages: 1) the Bag of Feature (BoF) approach, and 2) a decision-making model based on multiclass Support Vector Machine (SVM) classifier. The BoF is utilized for extracting the features from representative images. First, to provides excellent scale invariance break up the image into sub-regions by using speeded up robust features (SURF) detector. Then, compute the histogram of local detected features inside each sub-region. After that to create the visual words and to reduce the number of features, the K-means clustering approach is applied. The final sets of features are fed to a decision-making model based SVM classifier for automatic plant identification. Experimental results on several publicly available leaf datasets demonstrate that the effectiveness of the proposed method for plant classification compared to a set of state-of-the-art methods.
Publication Date
4-18-2018
Project Designation
Course Project
Primary Advisor
Russell C. Hardie
Primary Advisor's Department
Electrical and Computer Engineering
Keywords
Stander Symposium project
Recommended Citation
"An Efficient Leaf Recognition Based Approach for Plant Classification Using Machine Vision Strategy" (2018). Stander Symposium Projects. 1224.
https://ecommons.udayton.edu/stander_posters/1224
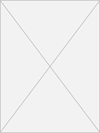
Comments
This poster reflects research conducted as part of a course project designed to give students experience in the research process.