Presenter(s)
Cameron E Long
Files
Download Project (11.2 MB)
Description
Recently the field of deep learning has made significant advancement, included in this category is the subject of sequence classification. Recent research has shown that traditional sequence processing deep learning architectures such as the Long Short-Term Memory cell (LSTM) or the Gated Recurrent Unit (GRU) can be replaced by Dilated Fully Convolutional Networks called a Temporal Convolutional Network (TCN). Other research has shown that by representing deep networks with complex and quaternion numbers, the networks tend to show improved convergence while using significantly less learned parameters. We combine these two observations to create a Quaternion Temporal Convolution Network (QTCN), and show its performance on sequential classification tasks.
Publication Date
4-24-2019
Project Designation
Graduate Research
Primary Advisor
Vijayan K. Asari
Primary Advisor's Department
Electrical and Computer Engineering
Keywords
Stander Symposium project
Recommended Citation
"Quaternion Neural Networks for Sequence Classification" (2019). Stander Symposium Projects. 1447.
https://ecommons.udayton.edu/stander_posters/1447
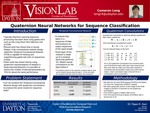