Presenter(s)
Wes Baldwin
Files
Download Project (2.6 MB)
Description
This poster presents recent work in the implementation of dimensionality reduction for neuromorphic camera data using time-surfaces. Neuromorphically inspired cameras can operate at extremely high temporal resolution (>800kHz), low latency (20 microseconds), wide dynamic range (>120dB), and low power (30mW). Time-surfaces are an ideal tool to leverage machine learning on event camera datasets as they assist in noise removal while retaining a high degree of spatial and temporal information. Combining time-surfaces with transfer learning is advancing state-of-the-art performance for object classification.
Publication Date
4-24-2019
Project Designation
Independent Research
Primary Advisor
Vijayan K. Asari
Primary Advisor's Department
Electrical and Computer Engineering
Keywords
Stander Symposium project
Recommended Citation
"Object Classification using Neuromorphic Cameras" (2019). Stander Symposium Projects. 1551.
https://ecommons.udayton.edu/stander_posters/1551
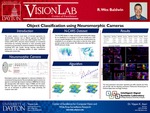