Presenter(s)
Jielun Zhang
Files
Download Project (697 KB)
Description
Network traffic classification is essential in network management and measurement in access networks, e.g., network intrusion detection, network resource allocation, etc. State-of-the-art Deep Learning based classifiers achieve high classification accuracy even when dealing with encrypted data packets. Such classifiers would need to be updated when a new application appears in the network traffic. However, it is challenging to build and label a dataset of the unknown application so that the current network traffic classifier can be updated. In this paper, we propose an autonomous model update scheme to (i) build a dataset of new application packets from active network traffic; and (ii) update the current network traffic classifier. In particular, the core of the proposed scheme is a discriminator includes a statistical filter and a Convolutional Neural Network (CNN) based binary classifier to filter and build a dataset of new application packets from active network traffic. Evaluation is conducted based on an open dataset (ISCX VPN-nonVPN dataset). The results demonstrated that our proposed autonomous classifier update scheme can successfully filter packets of a new application from network traffic and build a new training dataset. Moreover, the packet classifier can be effectively updated through transfer learning. The success of the proposed update scheme can be adopted in the access network for efficient and adaptive network measurement and management.
Publication Date
4-22-2020
Project Designation
Graduate Research
Primary Advisor
Feng Ye
Primary Advisor's Department
Electrical and Computer Engineering
Keywords
Stander Symposium project, School of Engineering
Recommended Citation
"Autonomous Model Update Scheme for Deep Learning-Based Network Traffic Classifiers" (2020). Stander Symposium Projects. 1843.
https://ecommons.udayton.edu/stander_posters/1843
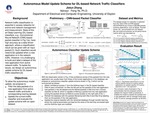