Presenter(s)
Md. Shahanur Alam
Files
Download Project (881 KB)
Description
Custom low power hardware systems for real-time network security and anomaly detection are in high demand, as these would allow for adequate protection in battery-powered network devices, such as edge devices and the internet of the things. This paper presents a memristor based system for real-time intrusion detection, as well as an anomaly detection based on autoencoders. Intrusion detection is performed by training only on a single autoencoder, and the overall detection accuracy of this system is 92.91%, with a malicious packet detection accuracy of 98.89%. The system described in this paper is also capable of using two autoencoders to perform anomaly detection using real-time online learning. Using this system, we show that the system flags anomalous data, but over time the system stops flagging a particular datatype if its presence is abundant. Utilizing memristors in these designs allows us to present extremely low power systems for intrusion and anomaly detection while sacrificing little accuracy.
Publication Date
4-22-2020
Project Designation
Graduate Research
Primary Advisor
Tarek M. Taha
Primary Advisor's Department
Electrical and Computer Engineering
Keywords
Stander Symposium project, School of Engineering
Recommended Citation
"Unsupervised Real-Time Network Intrusion and Anomaly Detection by Memristor Based Autoencoder" (2020). Stander Symposium Projects. 1849.
https://ecommons.udayton.edu/stander_posters/1849
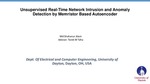