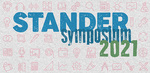
Data Analysis on Classifying the Severity of Genetic Mutations
Presenter(s)
Kelly Laureen Pleiman
Files
Description
Cancer tumors can have thousands of mutations but determining which of those mutations actually contribute to tumor growth is critical in understanding the disease. Through the use of productive models in machine learning, this capstone project focuses on determining the severity of different genetic mutations using available data from Kaggle on the mutation’s gene, variation, and clinical text evidence. By performing data analysis and applying different models on this complex data set, the class or severity of genetic mutations on a scale from 1-9 can be predicted. Decision tree, random forest, SVD, logistic regression, and K nearest neighbor are among the models that were used to classify genetic variation. Obtaining higher model accuracies allows for better classification of genetic mutations and could eventually expedite the time pathologists spend manually classifying mutations.
Publication Date
4-22-2021
Project Designation
Capstone Project
Primary Advisor
Ying-Ju Chen
Primary Advisor's Department
Mathematics
Keywords
Stander Symposium project, College of Arts and Sciences
United Nations Sustainable Development Goals
Industry, Innovation, and Infrastructure
Recommended Citation
"Data Analysis on Classifying the Severity of Genetic Mutations" (2021). Stander Symposium Projects. 2207.
https://ecommons.udayton.edu/stander_posters/2207
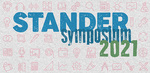