Presenter(s)
Supun Samudika De Silva
Files
Download Project (7.1 MB)
Description
Skin cancer is a cancer type with a very high mortality rate and an incidence rate. It is also a cancer type that is known to be treatable if detected early. However, the diagnosis accuracy of a human expert is highly dependent on their experience in visual inspection of skin pigmentation. An automated detection of skin cancer based on the analysis of an image of the suspected affected area would be helpful to physicians or dermatologists in order to present a fast and reliable diagnosis. Presently, Convolutional Neural Networks (CNNs) are one of the Artificial Intelligence techniques used widely for computer aided detection and diagnosis of skin lesions. In some cases, the images that are intended to be used towards training a CNN are preprocessed by segmenting the lesion area, correcting illuminations, applying color constancy, removing attention to artefacts around the lesion, etc. Dermoscopy images are a type of images that are being used with CNNs other than standard photographed clinical images. Most of the time, classification of the images is completely based on features generated using CNNs. Transfer learning is one heavily utilized approach that uses pre-trained networks that are mostly very deep and are able to be fine-tuned for skin lesion images to generate features. This presentation introduces common approaches followed to preprocess images and learning techniques that are used with CNNs followed by descriptions of two current methods that utilize CNNs to classify skin lesions for skin cancer diagnosis.
Publication Date
4-22-2021
Project Designation
Graduate Research
Primary Advisor
Russell C. Hardie
Primary Advisor's Department
Electrical and Computer Engineering
Keywords
Stander Symposium project, School of Engineering
United Nations Sustainable Development Goals
Good Health and Well-Being
Recommended Citation
"CNN-based Machine Learning Approaches to Skin Lesion Classification for Skin Cancer Detection and Diagnosis." (2021). Stander Symposium Projects. 2365.
https://ecommons.udayton.edu/stander_posters/2365
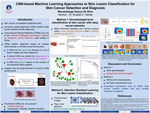