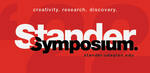
Anomaly Analysis in Medical Images
Presenter(s)
Kim Anh Phung, Nileshkumar Sadanand Wangad
Files
Description
Deep learning provides smart alternatives and efficient algorithms on data-driven models for data processing. The approach of learning from medical image data can deliver reliable findings and analysis which in turn can give us more accurate results in less time with low utilization of resources. Through neural network implementation, we can get much better results in classification which other methods fail to replicate. Deep learning based methods have shown to be beneficial in a variety of fields, and its application has aided us to consider the implementation of the COVID-19 epidemic. One challenging task observed is the recognition of COVID-19 symptoms. The most relevant type of detecting the symptoms is from human lung X-ray images without manual intervention. For this purpose, we implemented a novel algorithm to classify the positive epidemic cases from the dataset consisting of human X-rays labeled under covid and non-covid. The dataset is used for training through a series of different deep learning models to extract the feature and classify the images respectively, and then finally feed into a classifier to get the desired output. The training yielded an accuracy of 95% from this approach and can be considered for further implementation on different research topics in the near future.
Publication Date
4-20-2022
Project Designation
Independent Research
Primary Advisor
Van Tam Nguyen
Primary Advisor's Department
Computer Science
Keywords
Stander Symposium project, College of Arts and Sciences
United Nations Sustainable Development Goals
Good Health and Well-Being
Recommended Citation
"Anomaly Analysis in Medical Images" (2022). Stander Symposium Projects. 2470.
https://ecommons.udayton.edu/stander_posters/2470
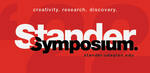
Comments
Presentation: 1:00 p.m.-1:20 p.m., Kennedy Union 211