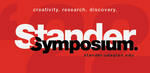
ReRAM In-Memory Computing for Online Reinforcement Learning
Presenter(s)
Md Shahanur Alam
Files
Description
Reinforcement learning (RL) has been examined to learn when an agent interacts continually with an environment to learn an optimal policy. Neuromorphic in-memory computing is a computing method that can be used to implement Artificial Intelligence (AI) on low power. Complementary-Metal-Oxide-Semiconductor (SRAM or DRAM) based in-memory computing systems have been developed for AI inference applications at the edge. These models are not able to perform on-chip training. Alternatively, significant progress has been made in Non-Volatile Memory (NVM) based systems that allow for on-chip training. The Resistive-RAM or ReRAM is an emerging NVM device, which has been examined for implementing in-memory computing systems in the analog domain. However, ReRAM neuromorphic systems have not been investigated extensively for the RL algorithm. This work presents a memristor crossbar circuit for on-chip reinforcement learning, where the learning process takes place in a dynamic environment. The success of learning is ensured by achieving the optimum average score of the agent in the presence of environmental variability.
Publication Date
4-20-2022
Project Designation
Graduate Research
Primary Advisor
Tarek M. Taha
Primary Advisor's Department
Electrical and Computer Engineering
Keywords
Stander Symposium project, School of Engineering
Recommended Citation
"ReRAM In-Memory Computing for Online Reinforcement Learning" (2022). Stander Symposium Projects. 2725.
https://ecommons.udayton.edu/stander_posters/2725
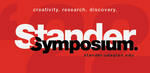
Comments
Presentation: 9:00 a.m.-9:20 a.m., Kennedy Union 311