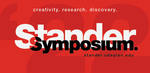
Exploration of Data Clustering within a Novel Multi-Scale Topology Optimization Framework
Presenter(s)
Kevin Robert Lawson
Files
Description
An advanced computational framework is needed to design next-generation aerospace structures capable of performing in increasingly extreme environments. Multi-scale topology optimization (TO) offers a solution in which a macroscale-level optimization conditions further optimizations at the mesoscale level, where designs for constitutive representative volume elements (voxels) are generated based on the properties called for at their location in the macroscale problem. As the desired properties of each macro voxel must be met through a unique, voxel-specific meso lattice architecture, the cost of large-scale design problems can be substantial. Aiming to increase efficiency without a significant loss in predictive fidelity, we explore the use of data clustering to reduce the number of targeted macro voxel properties and thus the number of homogenized meso lattice architectures needed to attain these properties. Four data clustering algorithms k-means, spectral clustering, DBSCAN, and OPTICS were implemented and gauged by their run time and variance from the unfiltered solution. A characterization of their performance reveals the most suitable grouping method and assesses the feasibility of clustering methods in a multi-scale TO framework. Preliminary results are presented for a three-point bend problem, which provides an ideal setting for experimental validation of the proposed computational methodology. With minimal variation from the optimized result, data clustering greatly reduces the computational cost of voxel design generation by lowering the number of unique designs. K-means clustering specifically has the lowest impact on the structural performance for a set number of groups, with a 97% reduction in voxel types with only an approximate 5% increase in compliance. The present work provides insight into how data clustering algorithms can be used to effectively pass data through a multi-scale TO framework, which will be particularly important as the framework evolves from a single anisotropic linear elastic material to multiple materials, inelastic deformations, and multi-physics loadings.
Publication Date
4-20-2022
Project Designation
Graduate Research
Primary Advisor
Robert L. Lowe
Primary Advisor's Department
Mechanical and Aerospace Engineering
Keywords
Stander Symposium project, School of Engineering
United Nations Sustainable Development Goals
Industry, Innovation, and Infrastructure
Recommended Citation
"Exploration of Data Clustering within a Novel Multi-Scale Topology Optimization Framework" (2022). Stander Symposium Projects. 2743.
https://ecommons.udayton.edu/stander_posters/2743
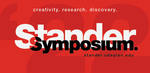
Comments
Presentation: 10:40 a.m.-11:00 a.m., Kennedy Union 222