Presenter(s)
Fangshi Zhou
Files
Download Project (1.2 MB)
Description
Recently variational autoencoders (VAE) have become one of the most popular generative models in deep learning. It can be applied to generate images, audio, text, and other data. We propose a novel parallel structure for Gumbel-Softmax VAEs, which combines m ≥ 1 parallel VAEs with different annealing mechanics for softmax temperature τ and adjusts τ at each training epoch based on the minimum loss from these VAEs. Our preliminary experiments demonstrate that our model with m > 1 (e.g., m = 5) outperforms the model with m = 1 in generative processes, adversarial robustness, and denoising.
Publication Date
4-19-2023
Project Designation
Graduate Research
Primary Advisor
Zhongmei Yao, Xin Chen, Luan Nguyen, Tianming Zhao
Primary Advisor's Department
Computer Science
Keywords
Stander Symposium, College of Arts and Sciences
Institutional Learning Goals
Vocation
Recommended Citation
"MinLoss-VAE: Min-Loss Parallel Variational Autoencoders with Categorical Latent Space" (2023). Stander Symposium Projects. 2888.
https://ecommons.udayton.edu/stander_posters/2888
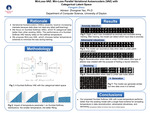
Comments
Presentation: 11:20-11:40 p.m., Jessie Hathcock Hall 180