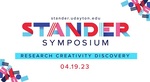
Prioritization of Discordant Chronic Comorbidities and prediction of its medication using machine learning
Presenter(s)
Ichchha Pradeep Sharma
Files
Description
About one in three adults worldwide suffer from multiple chronic diseases [1]. In the United States, about 12% of adults have five or more chronic conditions [2]. Such chronic conditions are increasing in the elderly, putting a high toll on our healthcare system. Patients with Discordant Chronic Comorbidities may require numerous appointments with general practitioners, complex and structured care, as well as enhanced coordination between various healthcare providers to ensure quality of care and treatment.With the large prevalence of multiple chronic conditions, it is difficult for the patients and providers to prioritize the medications and understand the impact caused by one treatment on the other simultaneous treatments. Healthcare practitioners trust the patients to actively participate in their care and treatment because of the prolonged nature of chronic disorders. Meanwhile, multiple factors affect a treatment, and it becomes difficult for patients to keep up with the interactions, change in body weight or the cost of a treatment. These factors need to be taken into consideration while making any recommendations.Numerous research studies are being done for managing and recommending medication for a single chronic disease. To the best of our knowledge, there are no solutions available to help people simultaneously manage their type-2 diabetes and any other disease. In this work, we seek to design a decision aid/recommendation system powered by state-of-art Machine learning algorithms to aid the healthcare practitioners and patients make informed decisions.We start out by designing a survey on Amazon Mechanical Turk. The survey captures multiple concerns and complex/changing needs of patients with DCCs. The goal is to use this survey to understand healthcare providers' treatment strategies for patients and how they prioritize the care of patients with complex care needs. Mechanical Turk provided us access to a diverse set of healthcare providers who have different experience and working in different contexts. After the collecting the data, it is cleaned to extract the required data for further processing. We tested out this data and are creating benchmarks using various state-of-art Machine Learning algorithms. Reference:[1] Hajat C, Stein E. The global burden of multiple chronic conditions: a narrative review. Preventive medicine reports. 2018 Dec 1;12:284-93.[2] Buttorff, Christine, Teague Ruder, and Melissa Bauman. Multiple chronic conditions in the United States. Vol. 10. Santa Monica, CA: Rand, 2017.
Publication Date
4-19-2023
Project Designation
Graduate Research
Primary Advisor
Tom Ongwere
Primary Advisor's Department
Computer Science
Keywords
Stander Symposium, College of Arts and Sciences
Institutional Learning Goals
Community; Diversity; Vocation
Recommended Citation
"Prioritization of Discordant Chronic Comorbidities and prediction of its medication using machine learning" (2023). Stander Symposium Projects. 2913.
https://ecommons.udayton.edu/stander_posters/2913
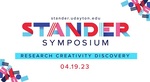
Comments
Presentation: 11:40 a.m.-12:00 p.m., Jessie Hathcock Hall 180