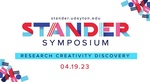
Predicting NBA Player Performance: An Analysis of 11 Seasons of Offensive Statistics
Presenter(s)
Payton Reaver
Files
Description
This project aims to utilize machine learning algorithms to predict a player's performance for the upcoming NBA season. The study utilizes a comprehensive data set comprising various offensive metrics. Such metrics are but not limited to: points, rebounds, assists, fouls, and blocks from the last 11 seasons of NBA basketball. By analyzing historical trends and patterns, this project seeks to develop a predictive model that can forecast a player's future performance accurately. The study has implications for fantasy basketball enthusiasts, sports analysts, sports betting, and team managers seeking to improve their decision-making processes.
Publication Date
4-19-2023
Project Designation
Capstone Project
Primary Advisor
Ying-Ju Chen
Primary Advisor's Department
Mathematics
Keywords
Stander Symposium, College of Arts and Sciences
Institutional Learning Goals
Vocation; Practical Wisdom
Recommended Citation
"Predicting NBA Player Performance: An Analysis of 11 Seasons of Offensive Statistics" (2023). Stander Symposium Projects. 2945.
https://ecommons.udayton.edu/stander_posters/2945
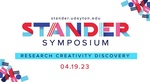
Comments
Presentation: 10:40-11:00 a.m., Science Center 119