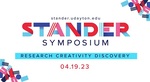
Seizure Identification and Prediction Using DeepLearning
Presenter(s)
Abigayle Hahn
Files
Description
Epilepsy is often characterized by uncontrolled electrical activity in the brain that manifests as seizures. Behavior has been used to identify seizure activity in humans for a long time and it is the most commonly used way to identify human patients with epilepsy. However, most of the behavioral scales that are used to identify seizures are observer biased and do not capture the dynamics of seizure activity. Importantly, we don’t have any reliable methods to predict the onset and severity of seizures. Recent advances in machine learning and artificial intelligence offer us new avenues to automate the identification of seizures from video datasets. We hypothesize that seizure activity in a preclinical mouse model of epilepsy can be reliably captured using a DeepLearning approach. Using a large dataset of clinically-relevant seizure activity in mice, we have annotated key anatomical features across different epileptic stages. We used the DeepLabCut toolbox to train a model to identify 28 key body components across our entire dataset. Our model is able to capture kinematic data in novel seizure videos with a high degree of accuracy. We plan to further refine the accuracy of our model using an active learning approach and by increasing the amount of training data. Using the kinematic data from our video data sets, we plan to identify and define seizure states from a purely data-driven standpoint. Our ultimate aim is to translate our approach to a clinical setting in order to prevent Sudden Unexpected Death in Epilepsy (SUDEP).
Publication Date
4-19-2023
Project Designation
Independent Research
Primary Advisor
Aaron Sathyanesan
Primary Advisor's Department
Biology
Keywords
Stander Symposium, College of Arts and Sciences
Institutional Learning Goals
Scholarship; Community
Recommended Citation
"Seizure Identification and Prediction Using DeepLearning" (2023). Stander Symposium Projects. 2987.
https://ecommons.udayton.edu/stander_posters/2987
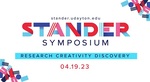
Comments
Presentation: 1:20-1:40 p.m., Science Center 146