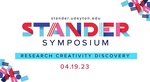
Analysis of IMDB Movie Ratings, with Emphasis on User Predictions and Recommendations
Presenter(s)
Reed Shay
Files
Description
The Movies Dataset, available on Kaggle, is a comprehensive dataset containing information on movies released between 1990 and 2017. In this project, we aim to analyze variables that have significant predictive power on the success of a movie and to build a recommendation system based on users' profiles.Firstly, we will explore the dataset and preprocess it to extract relevant information for our analysis. We will then perform exploratory data analysis to identify potential predictors of a movie's success, such as budget, genre, runtime, release date, and rating. We will use regression techniques to model the relationship between these predictors and a movie's success, as measured by box office revenue and user ratings. The results of our analysis will provide insights into the factors that contribute to a movie's commercial and critical success.In the second part of the project, we will develop a recommendation system that suggests movies to users based on their preferences. We will incorporate content-based filtering techniques, where we analyze the movie's features and recommend movies that are similar in genre, cast, or storyline to those the user has previously enjoyed. This can be a tricky problem as movie ratings are often times purely subjective and highly variable.Overall, this project will provide a comprehensive analysis of the movies dataset and a recommendation system that can help users discover new movies that match their preferences.
Publication Date
4-19-2023
Project Designation
Capstone Project
Primary Advisor
Ying-Ju Chen
Primary Advisor's Department
Mathematics
Keywords
Stander Symposium, College of Arts and Sciences
Institutional Learning Goals
Scholarship
Recommended Citation
"Analysis of IMDB Movie Ratings, with Emphasis on User Predictions and Recommendations" (2023). Stander Symposium Projects. 3165.
https://ecommons.udayton.edu/stander_posters/3165
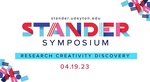
Comments
Presentation: 11:40 a.m.-12:00 p.m., Science Center 119