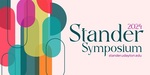
OptiVAE: A Unified Parallel Gumbel-Softmax VAE Framework with Performance-Based Tuning
Presenter(s)
Fangshi Zhou
Files
Description
Classic training algorithms for Gumbel Softmax Variational Autoencoders (GS-VAEs) often rely on an annealing scheme, which reduces the Softmax temperature according to a given function. We find that this leads to suboptimal performance. To improve the design, we propose a novel framework for GS-VAEs, which embraces dual latent layers and a parallel multi-model structure with diverse temperature strategies. By dynamically tuning the temperature in response to the loss difference between each sub-model and the best sub-model with the minimum loss at each training epoch, our model utilizes exploration and exploitation and significantly surpasses a standard GS-VAE in data reconstruction, detection of altered data, and model robustness. In particular, our model can reconstruct data of unfamiliar categories that are never observed during training. Moreover, in the presence of patch attack or white-box adversarial attack, our model greatly outperforms a standard GS-VAE and other existing models studied in this work.
Publication Date
4-17-2024
Project Designation
Graduate Research
Primary Advisor
Luan V. Nguyen, Zhongmei Yao, Tianming Zhao
Primary Advisor's Department
Computer Science
Keywords
Stander Symposium, College of Arts and Sciences
Institutional Learning Goals
Scholarship; Faith; Scholarship
Recommended Citation
"OptiVAE: A Unified Parallel Gumbel-Softmax VAE Framework with Performance-Based Tuning" (2024). Stander Symposium Projects. 3317.
https://ecommons.udayton.edu/stander_posters/3317
Comments
Presentation: 11:00-11:20, Kennedy Union 222