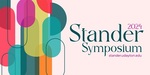
Design Space Exploration for a Novel Self-Healing Elastomer, Informed by Bayesian Optimization
Presenter(s)
Robert M. Drexler
Files
Description
Self-healing elastomers are an emerging class of materials capable of mitigating vulnerability to externally-induced damage. Recent advancements in polymer chemistry have led to self-healing elastomers that are 3D-printable, exhibit real-time self-healing in the absence of external stimuli (e.g., heat, light), and use commercially available (COTS) precursors to enable production at scale. However, at present, the trade-offs between virgin mechanical properties and self-healing efficiency are not well known. To address this research opportunity, this talk presents an experimental program – informed by a Bayesian optimization platform – to (a) facilitate design space exploration and (b) investigate the interplay between virgin mechanical properties (i.e., hardness and toughness) and self-healing efficiency (e.g., ratio of healed toughness to virgin toughness) as chemical composition is varied. The material of interest is BeckOHflex, a new acrylate/thiol-ene elastomer that exhibits real-time, autonomous self-healing and is exclusively prepared from COTS precursors. The experimental design was conducted by varying the crosslinker and thiol components from 0-10% by volume while holding the molar ratio of acrylate and photoinitiator constant. Test samples were cast in custom silicone molds and cured using an external UV lamp. Hardness data was obtained using an analog Shore OO durometer, and mechanical property data was collected through uniaxial tension testing. Informed by previous-iteration experimental inputs (chemical composition) and the resulting outputs from mechanical testing (virgin hardness, virgin toughness, and self-healing efficiency), a Bayesian optimization platform (EBDO+) was used to suggest next-iteration experimental inputs. Through this iterative process of synthesizing, testing, and analyzing different compositions throughout the experimental campaign, a well-defined Pareto frontier will be determined to bound the design space, allowing for a fundamental, quantitative understanding of tradeoffs between virgin mechanical properties and self-healing efficiency. It is expected that the Pareto frontier will be determined after tens of experiments out of a possible 2,000+ discrete input parameter combinations.
Publication Date
4-17-2024
Project Designation
Honors Thesis
Primary Advisor
Robert L. Lowe
Primary Advisor's Department
Mechanical and Aerospace Engineering
Keywords
Stander Symposium, School of Engineering
Recommended Citation
"Design Space Exploration for a Novel Self-Healing Elastomer, Informed by Bayesian Optimization" (2024). Stander Symposium Projects. 3375.
https://ecommons.udayton.edu/stander_posters/3375
Comments
Presentation: 2:00-2:20, Kennedy Union 310