Presenter(s)
Diya Liz Babu
Files
Download Project (206 KB)
Description
Predicting the performance of pilots in the aviation industry is essential for improving safety, efficiency, and overall operational effectiveness. Accurate evaluations of a pilot’s abilities can provide valuable insights for training programs, facilitate the development of customized training interventions, and contribute to the design of human-machine interfaces that align with individual skill sets. Moreover, understanding and predicting pilot performance can assist in identifying potential safety hazards and mitigating human factors that may contribute to errors in complex flight scenarios.This research primarily focuses on predicting and validating models for pilot performance during simulated flight operations, i.e. performing altitude or heading changes. This predictive scope involves essential metrics, the highest pilot entry stage input value (greatest yoke angle), aircraft heading error, aircraft heading change rate (yaw rate) during the pilot's initial recovery stage input, and compensatory corrections during the recovery period.Additionally, the research explores the temporal aspect of predictive accuracy, analyzing how early in the timeline we can effectively forecast pilot performance. Our proposed solution involves the utilization of Machine Learning Regression methods, assessing both Single Output and Multi Output models. Interestingly, the study reveals that Single Output models perform just as effectively as Multi Output models, suggesting a lack of correlation between the target variables for the Multi Output to outperform. Among the algorithms considered, Random Forest emerges as the most proficient in predicting pilot performance.
Publication Date
4-17-2024
Project Designation
Graduate Research
Primary Advisor
Van Tam Nguyen, Megan E. Reissman, Timothy Reissman
Primary Advisor's Department
Computer Science
Keywords
Stander Symposium, College of Arts and Sciences
Recommended Citation
"Pilot Performance Analysis in Virtual Environment" (2024). Stander Symposium Projects. 3417.
https://ecommons.udayton.edu/stander_posters/3417
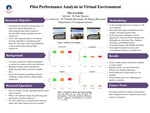
Comments
Presentation: 3:00-4:15, Kennedy Union Ballroom