Presenter(s)
Shruti Ajay Singh
Description
Artificial Intelligence (AI) has transformed today’s world with endless possibilities. We’ve reached a point where self-driving cars, and talking robots aren't science fiction anymore. Reinforcement learning (RL), a subset of AI, plays a crucial role in these advancements. However, as the lines between humans and machines blur, a question looms : “Can we trust AI to keep us safe and secure?”. RL unlocks the ability to learn on its own, but its learning can be manipulated making them vulnerable to adversarial attacks. Consider a self-driving car navigating a busy city street. Every lane change, signal interpretation, pedestrian interaction demands an instant decision in real-time. In an ideal world, the car receives noise-free sensory data, allowing the car to make safe decisions. However in a real-world scenario, the car is an easy target for malicious actors to manipulate the navigation system potentially leading to accidents. A threat that has severe consequences in other domains of RL applications : healthcare, transportation, finance. Therefore, achieving robustness against adversarial attacks requires a defensive framework tailored to the system’s characteristics. In this research, we address adversarial attacks on the observation state space in reinforcement learning. And we propose an entropy-based framework that detects and removes imposters by feature selection.
Publication Date
4-17-2024
Project Designation
Graduate Research
Primary Advisor
Luan V. Nguyen, Van Tam Nguyen
Primary Advisor's Department
Computer Science
Keywords
Stander Symposium, College of Arts and Sciences
Institutional Learning Goals
Diversity; Community; Diversity
Recommended Citation
"Feature Selection in Reinforcement Learning" (2024). Stander Symposium Projects. 3547.
https://ecommons.udayton.edu/stander_posters/3547
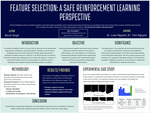
Comments
Presentation: 10:20-10:40, LTC Studio