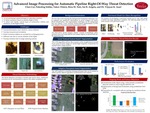
Advanced Image Processing for Automatic Pipeline Right-Of-Way Threat Detection
Description
Rapid advances made in the area of camera and sensor technology has enabled the use of video acquisition systems to monitor the right-of-way of pipelines. Huge amount of data is thus made available for analysis. However, it would be very expensive to employ analysts to scan through the data and identify threats to the right-of-way in the vast amount of wide area imagery. This warrants the deployment of an automated mechanism that is able to detect threats to the right-of-way and send out warnings in the event of detection of a threat. A novel algorithmic framework for the robust detection and classification of objects on pipeline right-of-way (ROW) is designed in four directions: visibility improvement, context-based segmentation, change detection, and part-based object recognition. In the first part of the framework, an adaptive image enhancement algorithm is utilized to improve the visibility of aerial imagery the can aid in threat detection. In this technique, a nonlinear transfer function is developed to achieve the enhancement process for the extremely non-uniform lighting conditions. In the second of the proposed scheme, the context-based segmentation is developed to eliminate regions from imagery that are not considered to be a threat to the pipeline. This segmentation algorithm allows to accelerate threat identification and improve object detection rate. Thirdly, a volumetric change detection algorithm utilizing dense point cloud representation flags changes in consecutive flights. The last phase of the framework is an efficient part-based object recognition model. This technique employs parts of the object with specific feature representative to characterize objects, which is robust to detect objects in partial occlusions and appearance variations. In other words, it is a stricter pre-trained classifier that searches imagery for specific targets that are considered threats. The classifier outputs location of threats and the severity of threat to pipeline.