Parameter Identification in Structured Discrete-Time Uncertainties without Persistency of Excitation
Presenter(s)
Ouboti Djaneye-Boundjou
Files
Download Project (270 KB)
Description
Concurrent Learning has been previously used in continuous-time uncertainty estimation problems and adaptive control to solve the parameter identification problem without requiring persistently exciting inputs. Specifically selected past data are jointly combined with current data for adaptation. Here, we extend the parameter identification problem results of Concurrent Learning for structured uncertainties in the continuous-time domain to the discrete-time domain. Alike the continuous-time case, we show that, in discrete-time, a sufficient, testable on-line and less restrictive condition compared to persistency of excitation guarantees global exponential stability of the parameter error when using Concurrent Learning.
Publication Date
4-9-2016
Project Designation
Graduate Research
Primary Advisor
Raul E. Ordonez
Primary Advisor's Department
Electrical and Computer Engineering
Keywords
Stander Symposium project
Recommended Citation
"Parameter Identification in Structured Discrete-Time Uncertainties without Persistency of Excitation" (2016). Stander Symposium Projects. 800.
https://ecommons.udayton.edu/stander_posters/800
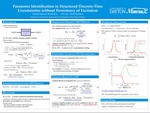