Presenter(s)
ALmabrok Essa Essa, Sidike Paheding, Daniel P Prince
Files
Download Project (1004 KB)
Description
Rapid advances in the area of sensor technology have enabled the use of video acquisition systems to create large datasets for analysis. However, processing big data requires extensive effort for human analysts. On the other hand, it is observed that many data, such as high-frame rate video, contain redundancy that cause extra work for analysis. Therefore, there is a need to develop an automated frame selection technique to reduce work load. In this research, we develop a method that can extract the most important and meaningful video frames from a large amount of data, while removing the insignificant ones to ease further analysis. These key frames can be selected based on the statistical analysis such as computing the mean and variance among a set of frames or between subsequent frames. We believe this technology benefits the computational performance of many real-world data processing systems, especially in current big data problems.
Publication Date
4-9-2016
Project Designation
Graduate Research
Primary Advisor
Vijayan K. Asari
Primary Advisor's Department
Electrical and Computer Engineering
Keywords
Stander Symposium project
Recommended Citation
"Frame Redundancy Elimination Technology for Big Data Analysis" (2016). Stander Symposium Projects. 832.
https://ecommons.udayton.edu/stander_posters/832
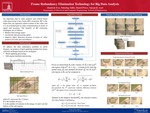