Presenter(s)
Barath Narayanan
Files
Download Project (1.0 MB)
Description
Early detection of pulmonary lung nodules plays a significant role in the diagnosis of lung cancer. Chest Radiographs (CRs) are currently being used by radiologists to detect such nodules. In this research, we present a novel cluster-based classifier architecture for lung nodule Computer Aided Detection (CAD) systems. We propose a novel optimized method of feature selection for both cluster and classifier components. For CRs, we make use of an independent database comprising of 160 cases with a total of 173 nodules for training purposes. Testing is implemented on a publicly available database created by the Standard Digital Image Database Project Team of the Scientific Committee of the Japanese Society of Radiological Technology (JRST). The JRST database comprises of 154 CRs containing one radiologist confirmed nodule in each. In this research, we exclude 14 cases from the JRST database that contain lung nodules in the retrocardiac and subdiaphramatic regions of the lung. Overall, with a specificity of 3 false positives per case/patient on average, we show a classifier performance boost of 7.7% for CRs when compared to a single aggregate classifier architecture.
Publication Date
4-5-2017
Project Designation
Graduate Research - Graduate
Primary Advisor
Russell C. Hardie, Temesgen M. Kebede
Primary Advisor's Department
Electrical and Computer Engineering
Keywords
Stander Symposium project
Recommended Citation
"Feature Selection Based Clustering Approach for Computer Aided Detection of Lung Nodules on Chest Radiographs" (2017). Stander Symposium Projects. 882.
https://ecommons.udayton.edu/stander_posters/882
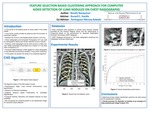