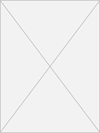
Data Mining of Smart WiFi Thermostats to Develop Multiple Zonal Dynamic Energy and Comfort Models of a Residential Building
Presenter(s)
Abdulrahman Mubarak Q Alanezi, Kefan Huang
Files
Description
Smart WiFi thermostats have gained an increasing foothold in the residential building market. The data emerging from from these thermostats is transmitted to the cloud. Companies like Nest and Emerson Climate Technologies are attempting to use this data to add value to their customers. This overarching theme establishes the foundation for this research. This research seeks to utilize WiFi data from the Emerson Climate Technologies’ Helix test house to: develop a dynamic model to predict real time cooling demand and then apply this model to running ‘what-if’ thermostat scheduling scenarios with the ultimate goal of reducing energy use in the residence or responding to high demand events. The Helix residence, with two thermostat controlled zones for each floor, exists in a temperature/humidity controlled external environment, which can be controlled to simulate environmental conditions present in the hottest to coldest climates. A Design of Experiment approach was used to establish data needed for the model. The control variables in the experiments included: levels for the exterior environmental schedule and levels for the interior setpoint schedules for both zones. Simply, this data enabled data collection for constant or cyclical exterior environmental conditions and constant and scheduled interior setpoint conditions, not necessarily the same for each floor. From this data, a regression tree approach (Random Forest) was used to develop models to predict the room temperature as measured by each thermostat, as well as the cooling status for each zone. The models developed, when applied to validation data (e..g, data not employed in training the model) R2 values of greater than 0.95. Then, the models developed were utilized for various ‘What if’ scenarios. Two such scenarios were considered. The first looked at the possibility of using the model to estimate comfort in a demand response event, e.g., when the grid manager calls for demand reduction. In this case, the heat pump providing cooling would be powered off for some time. The second scenario sought to quantify the cooling savings from use of higher thermostat setpoint during simulated non-occupied periods and for different exterior temperature schedules. The ‘What if’ predictions are validated with experimental data, thus demonstrating the value of the data-driven, dynamic data solely from smart WiFi thermostat information.
Publication Date
4-18-2018
Project Designation
Graduate Research
Primary Advisor
Kevin P. Hallinan
Primary Advisor's Department
Mechanical and Aerospace Engineering
Keywords
Stander Symposium project
Recommended Citation
"Data Mining of Smart WiFi Thermostats to Develop Multiple Zonal Dynamic Energy and Comfort Models of a Residential Building" (2018). Stander Symposium Projects. 1192.
https://ecommons.udayton.edu/stander_posters/1192
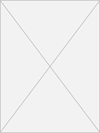