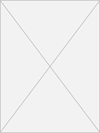
Multi-Integrated Segmentation Approaches for Permafrost Lakes Observed in Satellite Images
Presenter(s)
Ming Gong
Files
Description
During the summer melt season, permafrost lakes in the Arctic display a complex geometry. Casual inspection of remote sensing images shows that the lake phase of Arctic landscape undergoes a transition where disconnected lakes evolve into much larger scale connected networks with complex boundaries. Spatiotemporal dynamics of lakes is crucial for the stability of the Arctic climate system. To understand how these features evolve over time, we propose to develop two integrated machine learning image segmentation techniques for lake pattern recognition. Classical machine learning methodologies for image segmentation require handcrafted features that are similar to our visual perception and simple classification strategies to provide accurate boundaries. Conversely, deep neural networks for image segmentation learn these features through different variations of gradient descent to create these boundaries as well. The specific objectives of this research are to implement a classical image segmentation architecture and a deep convolutional encoder-decoder architecture called SegNet and apply each architecture to Landsat satellite imagery obtained from Google Earth Engine in 2016. The study area covers Siberia (both Western and Eastern), Chukotka and Alaska. We compare deep learning segmentation with classical segmentation methodologies for segmenting permafrost lakes to determine the capabilities of each methodology and their effectiveness for lake segmentation in a variety of Landsat imageries.
Publication Date
4-24-2019
Project Designation
Graduate Research
Primary Advisor
Ivan A. Sudakov, Vijayan K. Asari
Primary Advisor's Department
Physics
Keywords
Stander Symposium project
Recommended Citation
"Multi-Integrated Segmentation Approaches for Permafrost Lakes Observed in Satellite Images" (2019). Stander Symposium Projects. 1609.
https://ecommons.udayton.edu/stander_posters/1609
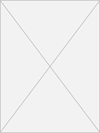