Presenter(s)
Dhaval Dilip Kadia
Files
Download Project (1.3 MB)
Description
The ultimate goal of science is a safer & healthier society and greater humanity. If the computer analyzes medical reports precisely, then enough time can be allotted to the individual patient; diagnosis can be accurate, time-efficient, cost-effective and labor saving. The objective of this research is performing 3-dimensional semantic lungs segmentation, by applying Deep Learning (DL) based methods on the sequence of Computed Tomography (CT) scan images. The motive is to design the 3-dimensional Neural Network architecture based on current 2-dimensional architecture, that is offering state-of-the-art performance, experimenting and evaluating it for improving its performance. The U-Net is a convolutional neural network that is a decent architecture for biomedical image segmentation, and applicable in volumetric segmentation. The proposed work will use the 3-dimensional patch in Recurrent Residual Convolution Neural Network (RRCNN) based U-Net (R2U-Net), applied on the sequence of CT scan images. These computational methods can replace the conventional methods, and overcome their limitations of time delays, the absence of a doctor, and unavailability of instruments. A large number of high-resolution CT images make numbers of slices, and some of the lesion features are not obvious, which leads the heavy work for doctors. The advantage of 3D imaging over 2D imaging is achieved by processing the higher dimensional data. 3D medical imaging can extract more features and surrounding information; that is helpful for the diagnosis. The output can be further helpful to recognize cancerous tumor with its volume inside the lungs. The proposed work will provide more opportunities to explore different modalities of medical imaging.
Publication Date
4-24-2019
Project Designation
Graduate Research
Primary Advisor
Vijayan K. Asari
Primary Advisor's Department
Electrical and Computer Engineering
Keywords
Stander Symposium project
Recommended Citation
"Multi-Dimensional Lung Segmentation using Deep Learning" (2019). Stander Symposium Projects. 1719.
https://ecommons.udayton.edu/stander_posters/1719
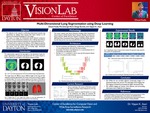