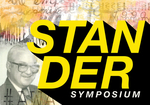
DALES: A Large-scale Aerial LiDAR Data Set for Semantic Segmentation
Presenter(s)
Nina Marie Varney
Files
Description
We present DALES, a new large scale aerial LiDAR data set with over a half-billion hand-labeled points, spanning 10 $km^2$ of area and eight object categories. Large annotated point cloud data sets have become the standard for evaluating deep learning methods. However, most focus on data collected from a mobile or terrestrial scanner with few focusing on aerial data. Point cloud data collected from an Aerial Laser Scanner (ALS) presents a new set of challenges and applications in areas such as 3D urban modeling and large scale surveillance. DALES is the largest publicly available ALS data set with over 400 times the number of points and six times the per meter resolution than other currently available annotated aerial point cloud data sets. We describe the nature of our data and the annotation workflow as well as provide a benchmark of current state-of-the-art algorithms and their performance on our data set. This data set gives a critical number of expert verified hand-labeled points for the evaluation of new 3D deep learning algorithms, helping to expand the focus of current algorithms to aerial data.
Publication Date
4-22-2020
Project Designation
Graduate Research
Primary Advisor
Vijayan K. Asari
Primary Advisor's Department
Electrical and Computer Engineering
Keywords
Stander Symposium project, School of Engineering
United Nations Sustainable Development Goals
Industry, Innovation, and Infrastructure; Sustainable Cities and Communities
Recommended Citation
"DALES: A Large-scale Aerial LiDAR Data Set for Semantic Segmentation" (2020). Stander Symposium Projects. 1939.
https://ecommons.udayton.edu/stander_posters/1939