Presenter(s)
Phillip Allen Clayton
Files
Download Project (431 KB)
Description
The pathway to sustainability is challenging. Multiple paths exist, but the key will be to achieve carbon reduction with the least cost. This could be achieved through large scale deployment of renewable energy; however, many studies have shown how important it is to reduce demand first. This study employs machine learning to analyze detailed energy profiles from the National Renewable Energy Laboratory (NREL), estimating potential energy savings for natural gas heating, electric heating, and electric cooling through modifications such as insulation improvements, setpoint changes, infiltration reduction, or system efficiency enhancements. By comparing these building models with actual building data from Cincinnati, Ohio, via a nearest neighbor approach, mean savings are calculated for the ten most similar simulated houses. This process allows for the use of limited data (annual energy usage for heating and cooling and house area) to identify comparable model sets and estimate potential areas for energy-saving improvements. When savings estimates vary significantly (coefficient of variation greater than 0.2), clustering is applied to find a more consistent subgroup, enhancing the accuracy of the energy savings predictions.This methodology proves particularly effective for high energy-consuming residences, which are often found within low-income housing sectors. By focusing on buildings with the highest potential for energy savings, this approach offers targeted insights for utilities and city planners looking to prioritize energy reduction initiatives effectively. It highlights buildings where interventions could have the most substantial impact, both in terms of energy savings and cost efficiency.The next steps will involve validating the estimated savings against actual data. This validation process is crucial for refining the methodology and ensuring its applicability and accuracy in real-world scenarios. Focusing on high-consumption, low-income buildings, this study aims to reduce energy demand, enhance sustainability, and help vulnerable communities achieve greater energy efficiency.
Publication Date
4-17-2024
Project Designation
Graduate Research
Primary Advisor
Kevin P. Hallinan
Primary Advisor's Department
Mechanical and Aerospace Engineering
Keywords
Stander Symposium, School of Engineering
Institutional Learning Goals
Scholarship
Recommended Citation
"Predicting Weather Dependent Energy Savings for Low-Income Residential Buildings for Specific Upgrades with Limited Building Data" (2024). Stander Symposium Projects. 3442.
https://ecommons.udayton.edu/stander_posters/3442
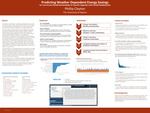
Comments
Presentation: 1:15-2:30, Kennedy Union Ballroom