Presenter(s)
Austin Christopher Grewell
Files
Download Project (36.3 MB)
Description
The approval procedure for new sustainable aviation fuels (SAFs) is a lengthy and costly process as it requires extensive testing. Several physicochemical properties must be measured for a new jet fuel, and their values must fall within specific required ranges. The ability to mitigate actual testing and measurements with predictive models would accelerate the certification process and reduce the associated costs. Machine learning (ML) algorithms are increasingly attractive tools for developing predictive models for the physicochemical properties of jet fuels.This project used machine learning methods to predict the density of hydrocarbon mixtures based on specific molecular descriptors. A dataset comprised of 17060 hydrocarbons with known density and corresponding molecular descriptors (group functionalities and topological indexes) was used to train ML models. Random forest and artificial neural networks were chosen as training algorithms. A hyperparameter optimization was used to determine the optimum parameters for each model.The random forest models trained with 80% of the dataset yielded R2 values greater than 0.98 for the remaining 20% of the dataset, indicating good performances and minimal to no overfitting. Models were tested on a set of random binary, tertiary and quaternary mixtures, yielding R2 values greater than 0.88.
Publication Date
4-17-2024
Project Designation
Course Project - MEE 460 01
Primary Advisor
Giacomo Flora
Primary Advisor's Department
Mechanical and Aerospace Engineering
Keywords
Stander Symposium, School of Engineering
Institutional Learning Goals
Scholarship; Critical Evaluation of Our Times
Recommended Citation
"Prediction of Hydrocarbon Density with Machine Learning Models" (2024). Stander Symposium Projects. 3649.
https://ecommons.udayton.edu/stander_posters/3649
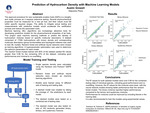
Comments
Presentation: 10:45-12:00, Kennedy Union Ballroom