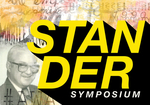
Semi Supervised Learning for Accurate Segmentation of Roughly Labeled Pathological Data
Presenter(s)
Rachel Rajan
Files
Description
Recent advancements in medical imaging research have shown that digitized high-resolution microscopic images combined with deep learning architectures have been able to generate promising results better than pathologists in the field of pathology diagnosis. But, for supervised deep learning techniques, the unavailability of labeled data has limited applications for accurate medical image segmentation. Hence, we propose an enhanced adversarial learning approach in semi-supervised segmentation for incremental training of our deep learning-based model to utilize unlabeled data in achieving better learning performance. Studies reveal that unlabeled data combined with small amount of labeled data can improve the overall performance considerably. Since most of the existing methods use weakly labeled images, our proposed technique utilizes unlabeled instances to improve the segmentation model. Experiments on two publicly available datasets such as PASCAL VOC2012 and UCSB Bio-Segmentation Benchmark dataset demonstrate the effectiveness of the proposed method.
Publication Date
4-22-2020
Project Designation
Graduate Research
Primary Advisor
Theus H. Aspiras, Vijayan K. Asari
Primary Advisor's Department
Electrical and Computer Engineering
Keywords
Stander Symposium project, School of Engineering
Recommended Citation
"Semi Supervised Learning for Accurate Segmentation of Roughly Labeled Pathological Data" (2020). Stander Symposium Projects. 1848.
https://ecommons.udayton.edu/stander_posters/1848